Industry 4.0 is more than just automation. Automation is a practice that has been around for decades since the first industrial revolution. Rather, as Roblek et al. (2016) rightly pointed out, Industry 4.0 is about the use of Internet of Things (IoT) to improve knowledge processes—how billions of data points can be located, acquired in real-time from machines and customers, shared, disseminated and collectively used by the different functions in the business to respond agilely to both internal and external gaps and opportunities.
1) Driving Productivity Gain with Reliable Real-time Data
One key productivity performance metric used for decades to identify hidden capacities and improve the productivity of operations would be the Overall Equipment Effectiveness (OEE). By breaking down and analysing OEE, manufacturers can easily pinpoint the different areas of losses (e.g. equipment breakdowns, material shortage, scrap parts and more). In practice, however, such critical information is often obscured by the manual way of data collection and ‘guesstimation’ of downtime figures and reasons.
In order to improve the accuracy and reliability of OEE as well as to obtain more insights for productivity improvements, manufacturers looked towards the use of Industry 4.0 technologies. Through the use of sensors and/or IoT devices, manufacturers can now automatically collect quasi-real-time data points at high sample rates such as output per minute and speed losses from machines, production lines and even the entire production floor. Such information cannot be tracked by manual data collection as they frequently occur throughout the day, and at short durations.
On an immediate basis, production supervisors can make data-driven performance improvements based on OEE and other insights displayed on the dashboards. For example, the dashboard could highlight the critical bottleneck equipment that has to be managed or flag out exceptionally high assembly times per step that should be looked out for. Production supervisors can also identify the top OEE losses such as changeovers and the wait for materials which they were previously oblivious to. With the duration of each event tracked and reflected in real-time, production supervisors know exactly which are the most pressing issues and focus resources on tackling the most critical issues. Finally, the performance gap between different shifts or production lines can also be identified and analysed in real-time.
Figure 1. Trend Analysis using Auk Industries’ platform
Given more time and data points collected, the big data analytics systems can leverage on its Artificial Intelligence (AI) function to optimise scheduling and load balancing for maximum productivity. For example, AI can sift through many variables such as job urgency, operator skills set, the equipment required and their differing setup times and run rates etc. to propose a schedule that increases resource utilisation while reducing costs. Moreover, AI could also take into consideration real-time and historical data to suggest the most optimal load balancing strategy. While it may be possible to do so via human resources, it is much time-consuming and human biases may come into play to render the strategy less effective.
2) Pioneering More Effective Maintenance
An increasing number of maintenance engineers are moving away from breakdown and time-based maintenance to usage-based and/or condition-based maintenance. Compared to the former, the latter allows them to call for maintenance and repair the machine ahead of a breakdown, thereby significantly reducing downtime.
The fastest and easiest way to implement usage-based maintenance is to use Current transformers (CT) sensors to track machine run-hour. CT sensors are non-invasive sensors which are affordable, readily available and can be deployed within minutes to track the motor or equipment run-hour. Notifications can be triggered and sent when the run-hour exceeds the recommended duration.
“CT sensors and usage-based maintenance often go hand-in-hand. Clients often start with CT sensors in their first phase of digital transformation because of its ease of deployment.
In the subsequent phases of digital transformation, users can leverage Industry 4.0 technologies to further improve their maintenance regime by engaging in condition-based maintenance. With IoT devices, critical machine/equipment parameters of both new and ageing equipment can be monitored in real-time, round the clock – this is akin to a 24/7 ‘health check-up’ for the various equipment. Examples of the parameters that can be tracked would include motor speed, current consumption pattern, vibration and surface temperature. When an anomaly among the parameters occurs, the relevant personnel can be notified immediately and make rectifications before an unplanned breakdown happens.
3) Reimagining Quality Management
To establish long-term competitiveness and sustainability, maintaining processes quality and ensuring quality management is vital. With the advent of Industry 4.0 technologies, manufacturers are able to gather more measurement data than before and detect defects that would otherwise go unnoticed by manual quality inspections.
There are three popular ways of ensuring quality. The first is the use of process capability to ensure product quality. By integrating real-time data with pre-built Statistical Process Control (SPC) modules within real-time monitoring systems, the Quality Control and Production Engineers can easily monitor the Process Capability Index (Cpk) from the dashboard, or toggle between the control charts and yield trends to manually spot any special cause variation. Whenever a performance deviation is detected, the relevant stakeholders would be alerted and implement corrective actions to address such nonconformities issues.
Finally, there is predictive quality analytics where statistical algorithms such as regression and time series analysis are applied to aggregated data to identify patterns and predict future anomalies or errors. By having these information, production managers can pre-empt and control potential product quality and safety issues, thereby reducing the likelihood of delivering poor-quality products to the end customers.
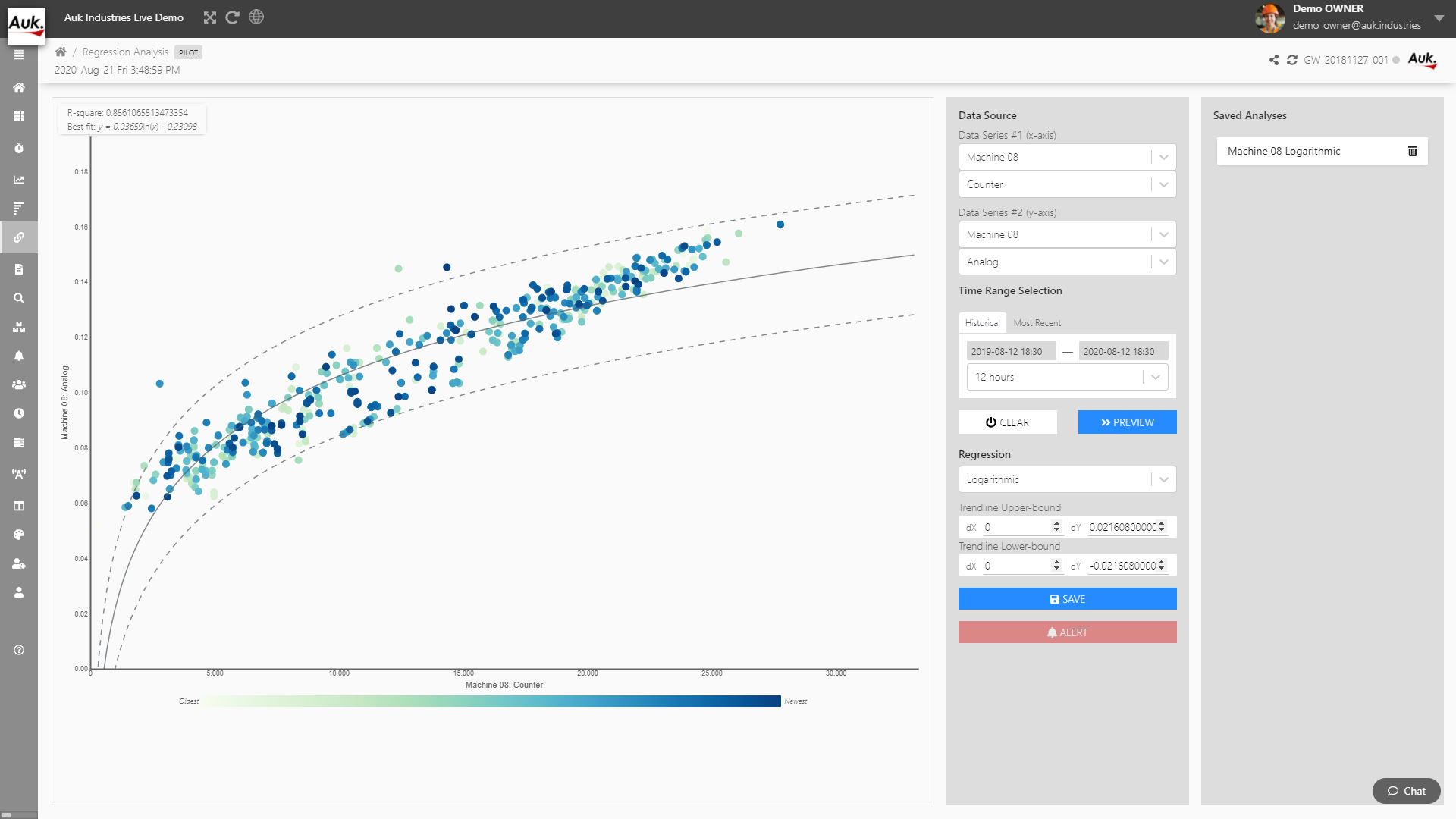

4) Realising Energy Management and Consumption Savings
Propelled by the growing emphasis on sustainability, legislatures, rising costs, and business continuity, energy management and efficiency in the factory has never been more important.
One of the ways to maintain efficient and effective consumption of energy would be to take action based on the insights derived from Industry 4.0 energy management systems (EMS). For a start, the EMS must be automatically fed with the IoT-collected machine data. This can be done via the cloud for a hassle-free experience. After which, the EMS can help to identify and quantify energy losses incurred across different batch processes. This is achieved by analysing OEE with other data such as process cycle time, and energy consumption. The EMS is also capable of constantly monitoring factors like applied power and harmonics which often cause power interruption, unnecessary downtimes and safety issues. Given these insights, manufacturers can then prioritise and decide on the most suitable energy management strategies to seek improvements.
In the event that manufacturers only require a simple energy monitoring system to track energy consumption patterns, across different time and segments of the production floor, there are less complex, easier to understand and affordable options available. For one, they may consider investing in simpler systems such as general power meters that can be purchased off the shelves and installed onto the main/sub switchboards or machine control panels. The data is then collected by a universal IoT device and sent to a visualisation platform.
Conclusion
Considering the global seismic shift towards digitalisation, the adoption of Industry 4.0 technologies among manufacturers has been accelerated. While the early adopters are already reaping the benefits of Industry 4.0 as shown in the above use cases, there are still many opportunities for the remaining manufacturers to capture. Now is the time to get started before one becomes a laggard.